Case Study: The Fatty Liver Foundation Develops an AI Risk Stratification Tool
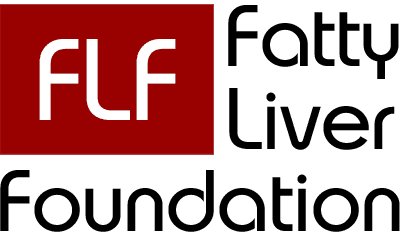
Nearly one in three Americans has a fatty liver. Most of them don’t even realize it.
Nonalcoholic fatty liver disease (NAFLD) is largely asymptomatic until it progresses to its later stages — which include nonalcoholic steatohepatitis (NASH), an often life-threatening condition that may progresses to cirrhosis. NASH is considered to be a primary driver of liver cancer and is one of the leading causes of liver cancer among liver transplant candidates in the United States.
The Fatty Liver Foundation (FLF), a leading patient-focused non-profit organization, is teaming up with Array Insights to help increase awareness of fatty liver disease so patients can act before it’s too late.
Partnership Goal
The AI Fatty Liver Risk Stratification Tool is designed to help accelerate FLF’s mission to identify asymptomatic, undiagnosed patients with liver fibrosis or early cirrhosis caused by fatty liver disease. It was especially important to provide a tool that could be easily accessible for patients and their care providers.
The Bottom Line
FLF and Array Insights are joining forces to use machine-learning technology to help patients and their primary care physicians assess risk for NAFLD and NASH.
The partnership has produced the AI Fatty Liver Risk Stratification Tool. This tool aims to help patients assess their risk factors for fatty liver disease and educate them on the necessary lifestyle changes to halt or minimize disease progression. Pharmaceutical and research organizations will also be able to use this tool to more effectively identify patients for clinical trials.
Early results show that the tool, which is expected to be available for use in early 2024, has registered a 77% detection rate for NAFLD.
3 Challenges: Building awareness around fatty liver disease risk
FLF’s chief goal is to improve the lives of both asymptomatic and diagnosed patients of fatty liver disease. A major step in achieving that goal is improving the medical community’s ability to identify and diagnose conditions at the earliest stage possible. In pursuing this objective, here’s what FLF and Array Insights encountered in this journey:
1 Inadequate Screening of At-Risk Populations.
NAFLD is closely tied to certain metabolic conditions and lifestyle factors. Those particularly at risk include individuals with type 2 diabetes, obesity and metabolic syndrome. These syndrome encompasses conditions such as high blood pressure, elevated fasting blood sugar levels, excess body fat around the waist, high triglyceride levels and low levels of high-density lipoprotein (HDL) cholesterol. However, because fatty liver disease often doesn’t exhibit early symptoms, it’s frequently overlooked.
One of FLF’s key strategies is to encourage physicians and patients to screen for asymptomatic liver disease in those at risk for the above conditions.
2 Need for Common Understanding Between Patients and Physicians.
Not all primary care doctors fully comprehend the complexities and risks associated with fatty liver disease. This gap in understanding can lead to missed opportunities for early diagnosis, proper referral, and intervention. FLF recognized the need to foster a shared understanding of these conditions among both healthcare providers and patients. Such an understanding includes recognizing the likelihood that a patient’s conditions will increase in severity and establishing frameworks for when more advanced testing is warranted.
3 Challenge in Finding the Right Machine Learning Expertise.
While FLF had strong ties to important patient and physician communities, it lacked in-house data science expertise. Analyzing large volumes of data would enable FLF to create more robust resources for screening and risk stratification. Before engaging with Array Insights, FLF had not identified a data partner it could endorse.
3 Solutions: The FLF & Array Insights Strategy
FLF and Array Insights worked together to design the AI Fatty Liver Risk Stratification Tool. Their gameplan included three key steps:
1 Combine Deep Data Expertise with Robust Community Relationships.
FLF’s network includes thousands of patients, physicians and other key stakeholders in the fatty liver community. Array Insights brings collaborative health data research software and data science expertise. Both halves came together to set a lay the groundwork for the project; FLF took on the role of raising awareness and connecting with healthcare institutions for data access, while Array Insights focused on building the technology.
2 Utilize FLF’s Access to Vital Patient Data.
As a patient advocacy organization, FLF was already stewarding a robust set of patient data from their Screening for Undiagnosed NAFLD NASH (SUNN-1) study. Array Insights was able to train its advanced machine learning models on this data to start building the groundwork for a screening tool to risk stratify patients who were concerned for their health.
3 Implement Patient-Centric Privacy from the Basic Design.
The Array Insights team employed a pioneering form of patient-centric AI technology — machine learning and analytics on federated data — to ensure that all fatty liver patient data stays contained, and all data uses are loggable and auditable. The model ensured that patient data could not be copied and pasted, emailed, forwarded, downloaded or found on unauthorized servers. This approach allowed for integration with large medical records systems while still ensuring that patient privacy remained fully protected.
Partnership Impact
During the initial testing phase, the tool achieved a 77% detection rate for NAFLD. FLF and Array Insights have plans to further train the tool’s machine-learning model with additional patient data to continue building validation. FLF and Array Insights anticipate that the risk stratification tool will be available for public use by early 2024.
“As someone diagnosed with cirrhotic NASH, I understand the dangers of fatty liver disease — and the steps needed to keep this condition from progressing. The risk stratification tool we are developing with Array Insights can help patients identify their risk and more quickly seek lifestyle changes and treatments, if needed. We hope that the development of increasingly useful ML tools, such as those that can give patients greater insight into their health and be used by physicians, can begin to erode the barriers that now impede improvements in patient wellness.”
Is your group looking to explore machine learning and AI data solutions?
Schedule a call with the Array Insights team to learn how your patient-centric organization can accelerate your research goals with technology that puts patient privacy first.